Traditional neuroscience has reached an impasse, a plateauing in understanding. Mainly relying on technological advancements to further its understanding of the brain’s neurology, it is now severely lacking in theory. Pure data gives little insight without theory, yet neuroscience struggles with theory generation in its traditional framework. Top-down explanations from computational models and mathematical models may help us produce some intuition. Prediction processing does this and more. It unifies all of the brain under essentially one function—prediction.
Philosophical Problems of Traditional Neuroscience
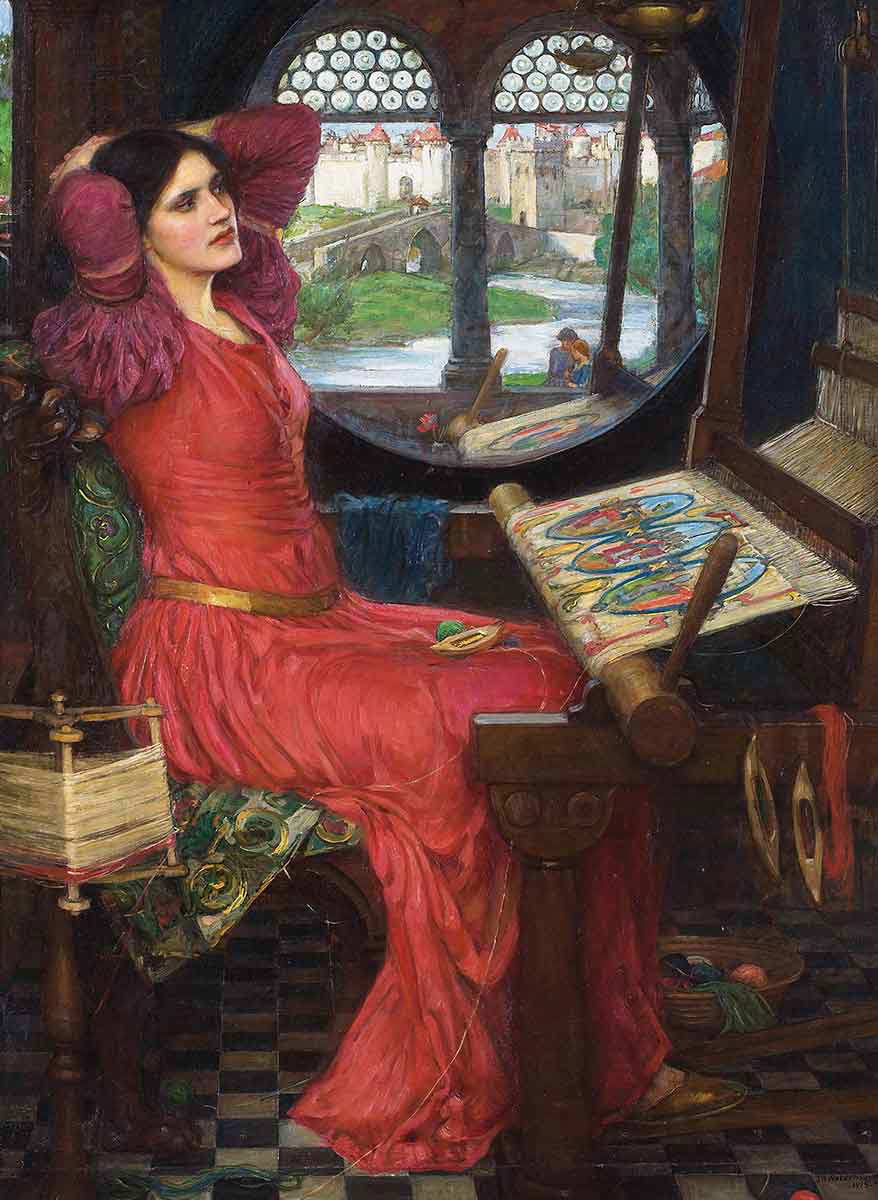
A major philosophical problem in brain science is how to integrate inherently reductionistic neuroscience with higher-level explanations of the brain and behavior, like psychology and cognition. Even if these were just folk psychology concepts, it is unlikely that we could eliminate them. Brain imaging mapping locations of activity to functions has proven to help little to bridge the gap and has shown itself to be poor in producing theories.
Even if we could completely split the brain into modules and functionally explain them separately, there would still be a lack of a unified explanation. How do the functions integrate and operate together? This integration problem operates on all levels. How do the descriptions of one level give us an explanation of the larger complex system?
Computational Neuroscience
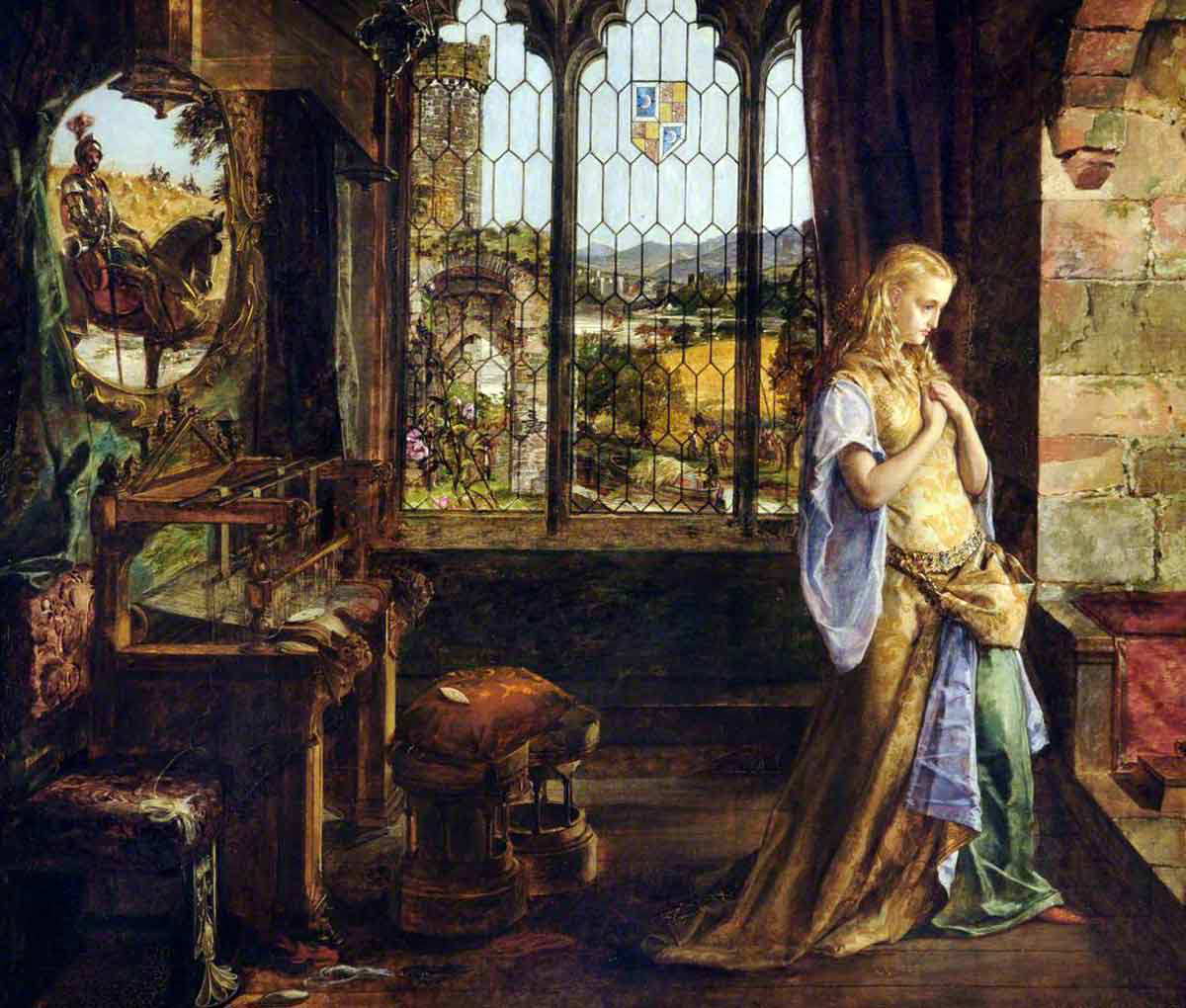
For this reason, Neuroscience has increasingly turned to computational neuroscience, which employs computational simulations to understand neuroscientific principles. This allows you to describe and explain it mathematically, and with simulations, you allow for testing and potential integration with other fields that can employ these models and algorithms.
This has led to frameworks and models trying to emulate certain parts of the neuronal system, like connectionism, neural networks, or dynamism with its nonlinear dynamics. These frameworks still struggle with vertical explanations. Their mechanisms do not intuitively lend themselves to coevolution, even if they would be good models of the brain.
Predictive processing is such a mathematical model that tries to explain what the system is trying to do, from the lower to the higher levels—which may explain why it is a new darling of neuroscience.
Top-Down and Bottom-Up: History of Inference
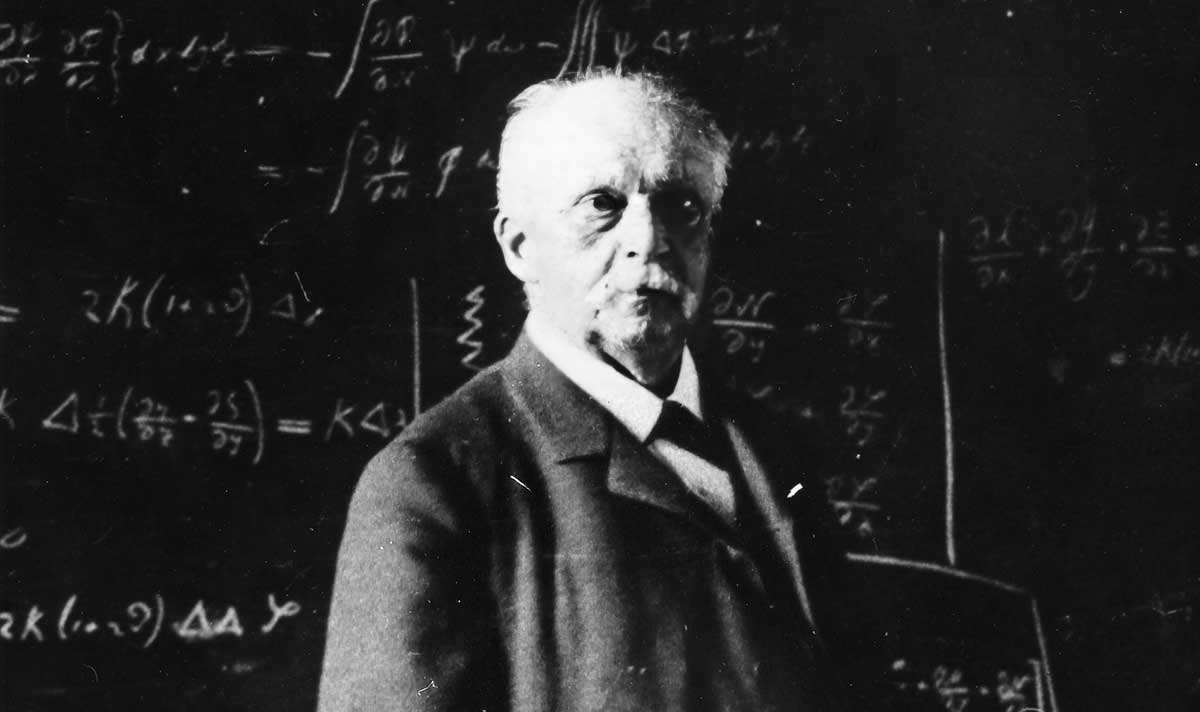
To understand predictive processing, it makes sense to examine perception, the field for which it was first developed.
One of the core questions of perception in neuroscience underpinned this development: whether perception is top-down or bottom-up, whether the brain generates at least parts of the world first, or if perception is mainly data-driven.
The importance of top-down processing has been a consideration since the time of Ibn al Haytham, but was most recognizably brought up by Kant, “The understanding can intuit nothing, the senses can think nothing. Only through their unison can knowledge arise“ (Critique of Pure Reason, 1781; 1787, A50-51/B74-76).
The primary influence for the modern inferentialist account is Helmholtz, who took Kant’s idea and employed it in his testing of the brain. He shaped a framework of perception driven by a hypothesis-driven brain, using unconscious perceptual inference from prior learning to perceive.
Karl Friston matched Helmholz’s idea with Bayes’s statistical approach. Something which fit it very well due to Helmholtz’s inference already had a Bayesian character in that it weighs alternatives and accounts for strong and weak evidence in its inference. Predictive processing is a Bayesian inferentialist model of perception; the brain infers or predicts the world state before the sensory input, making it a top-down generative model.
The Traditional Approach
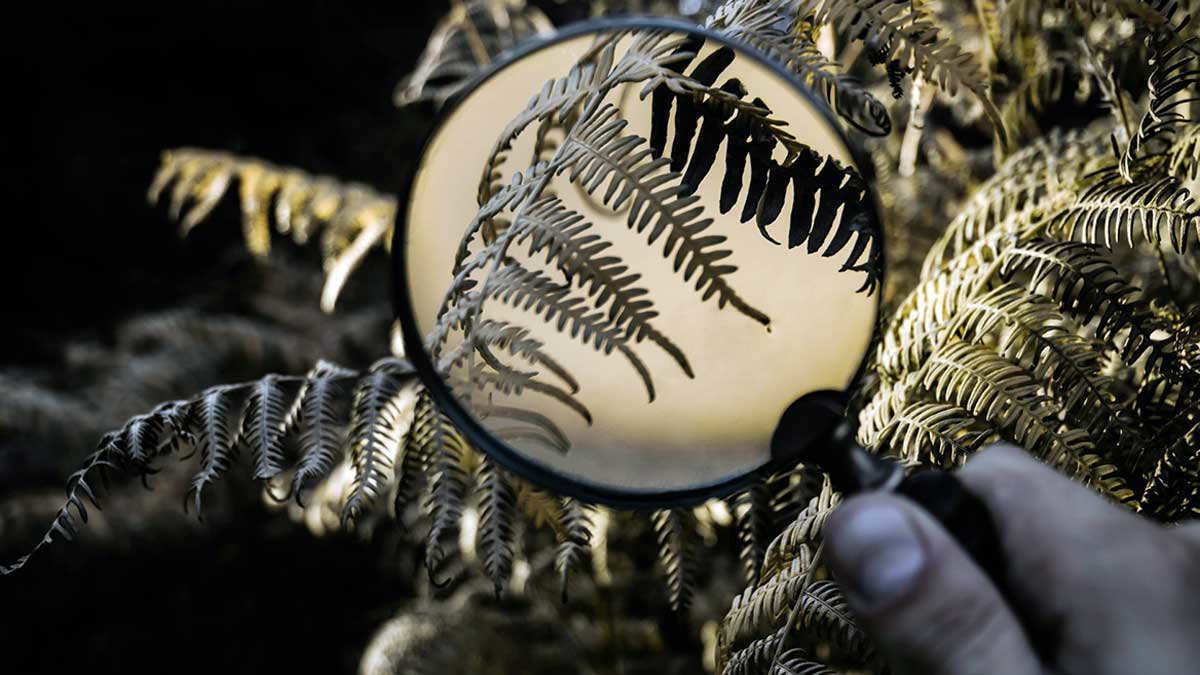
In contrast, the traditional neuroscientific theory—feature detection theory—is bottom-up, which takes perception to be built up from the input, with the detection of simple features combined to construct more complex representations.
The problem with this classical account is that little is understood about how this feature integration happens; it also struggles to explain context and other modulations of perception.
A related perceptual problem for bottom-up is the inverse problem—since the brain does not have direct access to the external world, the data does not specify the cause of the stimulus, yet knowing the cause will change our perception. Perception needs prior knowledge in terms of contextual cues to be understood.
The benefit of a top-down model is that it is baked into it inherently. This makes both feature binding and the inverse problem a non-issue.
A Closer Look at Vision
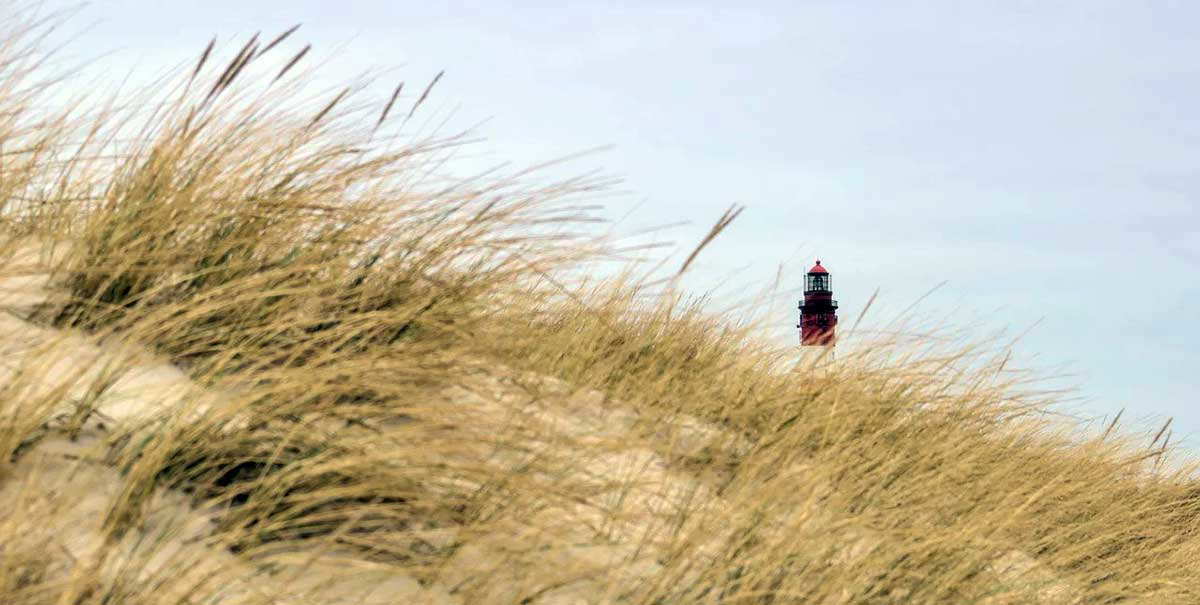
It can be clearly shown that perception is modulated by top-down processes by our capability to be fooled. What may look like a person standing on a cliff might be a lighthouse on a cliff further away. The perception changes with additional knowledge, indicating a lighthouse or a second cliff, and one can recognize the distance or the stimuli. If we just took the world as it is, this shift—like a visual illusion—would never occur.
This phenomenon can be captured by prediction coding, a computational model first developed for neuroscience by Rao and Ballard (1999). It is influenced by a model of visual compression, which states that if you know the properties of one pixel, you can accurately predict the properties of those surrounding it.
Prediction coding employs a generative model of the world and a hypothesis—a prediction of the incoming stimuli. It uses the difference between the predicted and actual stimuli to produce a prediction error, which is used to update the model.
Based on the context of a nearby cliff and the then-perceived distance of the object, the inference was made that it was a human, based on the knowledge of them having an ambiguous shape at a distance. With the additional context of a second cliff behind it, the hypothesis shifts to it being a lighthouse; even though the image is the same, based on the new information on depth and location, inferring it as a lighthouse makes more sense.
More simply, the model compares the different hypotheses and their probabilities for being the hidden causes of the stimuli based on continuously updated evidence. Prediction allows us to reduce the bandwidth of perception, process faster with context, and even react to things we wouldn’t have enough time to process normally.
Minimizing Prediction Error
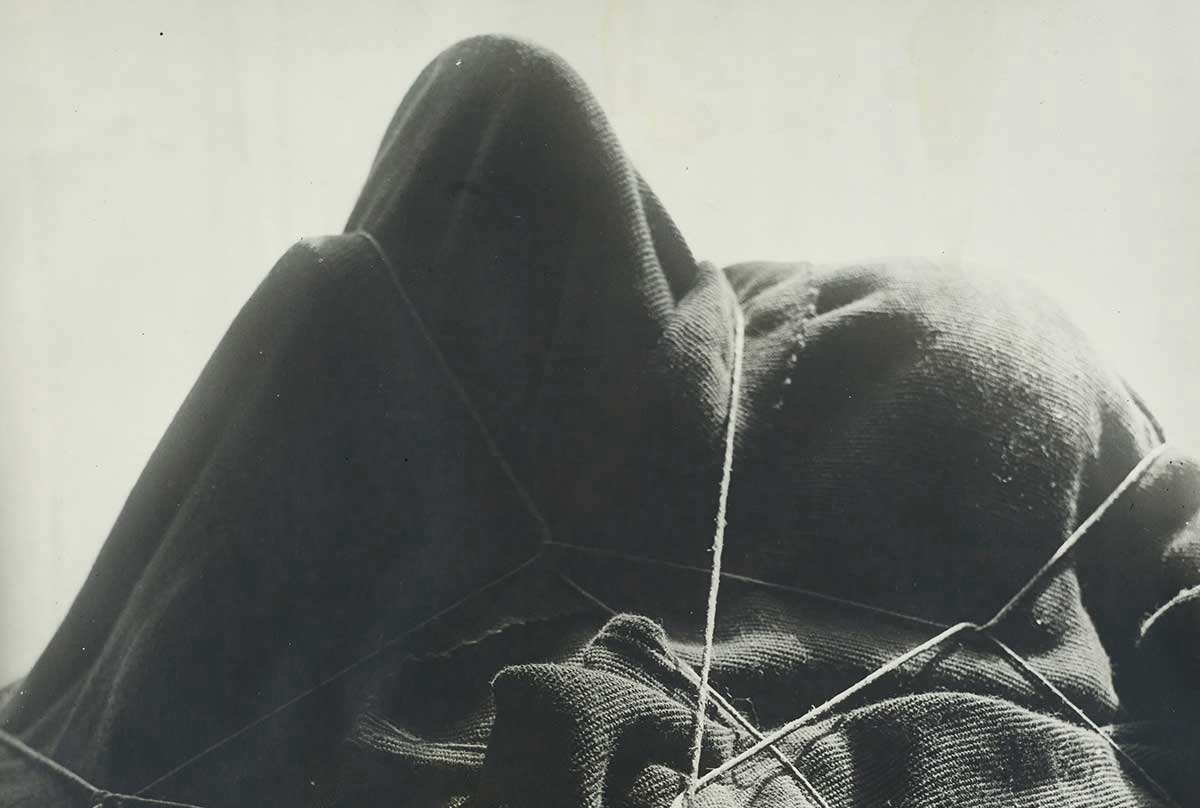
Generalizing this model, we can say that it minimizes prediction error. This can be on a short time scale, but especially on a longer time scale. The model tries to approximate the real world from its limited information according to its own requirements.
To further this aim, precision is also needed. It assesses how noisy the incoming input is, weighs how much its evidence should update the generative model, and determines how precise the corresponding prediction error is. It assesses its reliability, which can be extended over the long run, making it a factor in terms of predictions as well.
Just as updating the model based on unreliable data is unwise, following unreliable predictions is also unwise. Thus, the model checks prediction precision and prediction errors. The higher the precision, the more stable the hypothesis and the stronger the impact of evidence.
A demonstration of this concept is that we have been shown to predict the taste of wine based on its color—a red wine with a white wine color can be perceived to taste like a white wine (Morrot, Brochet, and Dubourdieu, 2001). This is due to experience; the hypothesis that white wine tastes like white wine has very high precision and thus is stubborn to changes from data.
While this might also come from just how close to each other the two tastes are, other examples are visual hallucinations in those without delusion disorders; we tend not to update our world model based on these, however real they appear to be and however consistent, like in Charles Bonnet Syndrome.
Active Inference
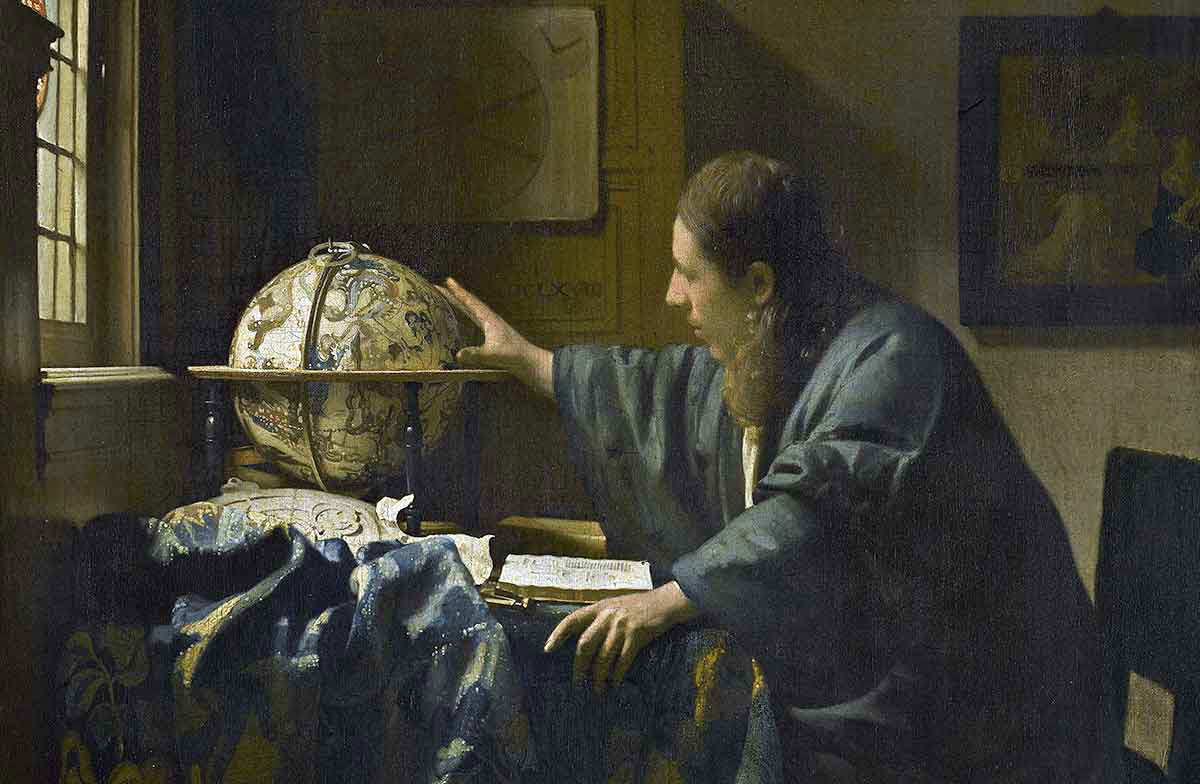
Minimizing the prediction error in terms of its interoceptive and exteroceptive perception aims to maintain the expected state, its homeostasis. For a self-corrective organism to maintain it, it requires action.
The inference framework can be extended almost directly to action. In active inference, action also minimizes prediction errors.
Low blood sugar leads to an interoceptive prediction error, and an update of top-down expectations causes hunger. Hunger causes a prediction error resolved through autonomic feedback, metabolizing fat, and guiding allostatic action toward fixing it.
Action is also a way of sampling the world, something that is needed to minimize prediction error. The more precise the data, the better. Most information we gain is through intervening with the environment as well. Hearing a sound, we typically try to find out what made it by scanning the environment, trying to find likely culprits. A certain sampling of evidence requires action to obtain, like feeling the texture of something, assessing the depth, or finding causes.
In active inference, to actually initiate an action, a certain state is predicted. The easiest way to minimize its prediction error and uncertainty is to initiate the action. Planning can be done by trying to move towards optimality in terms of long-term error minimization.
Two Roads of Understanding
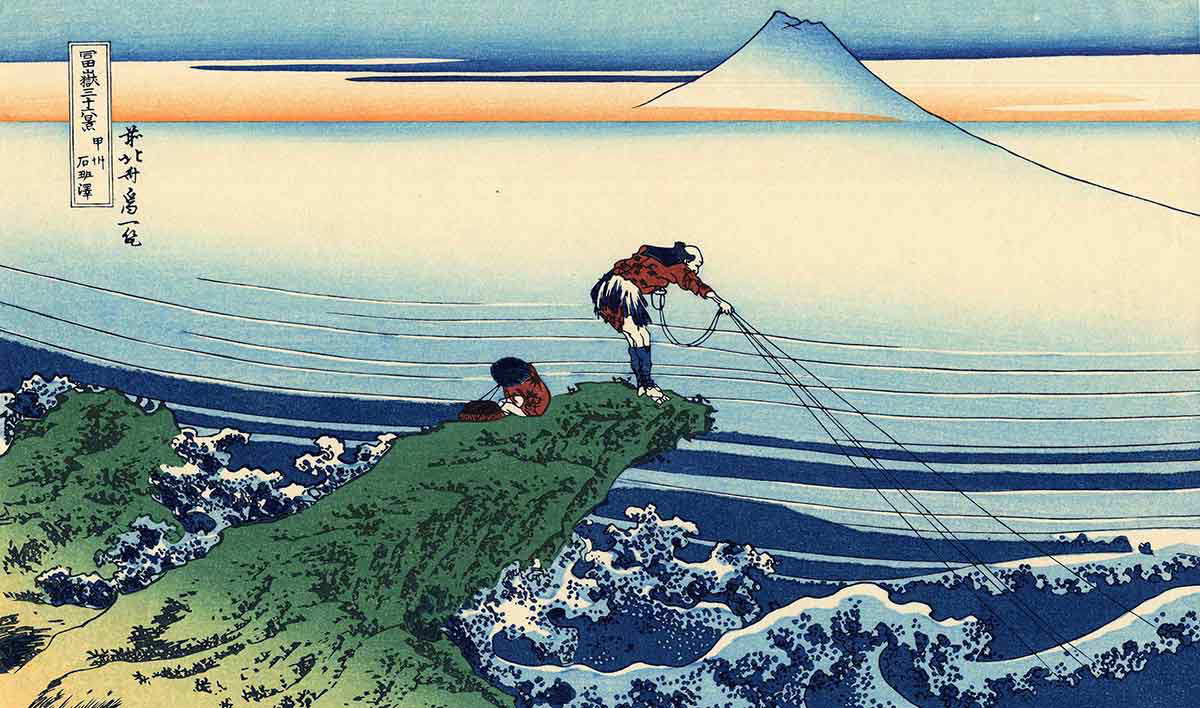
Now, one could stop here and implement this well-developed framework of action and perception and employ it with other frameworks in the brain to explain different functions—a pluralistic view, like Andy Clark proposes.
Karl Friston, the primary developer of this framework, has extended prediction error minimization (or free energy, as he denotes it) to be the basal process of any system—from particles to us. Focusing on us, it says that, as a living system, we try to maintain our preferred states by minimizing prediction error between them and the actual state. This truly unifies the brain, but it also extends us as a complete biological system.
On either road, one takes this framework for understanding that the brain is currently very flexible, even while still being unified. It combines already existing neuroscience frameworks, like embodiment and enactiveness and specific models of attention, and crafts new ones for areas like consciousness. It can cut through most dialectic issues of cognitive neuroscience like brain-mind, brain-body, and representationalism-emergentism.
Practicing brain scientists have extensively employed this predictive processing framework, and the results have largely supported the notion of the brain as a prediction machine in areas of perception and action. It is also being used to try to explain disorders like schizophrenia and autism.
This is not to say that it doesn’t have problems. Predictive Processing under its current parameters has been shown to be computationally intractable, meaning it would be physically impossible for the brain to perform such processing.
Predictive Processing is also just the most well-known model of us as prediction machines or inference engines. The Bayesian brain hypothesis is the larger idea of basing brain processing on Bayesian probability theory.
This framework has wide-reaching effects on the philosophy of mind, and accepting its premises influences how we see consciousness, free will, epistemology, and reality. But it bears mentioning that it is just that, a framework to model the brain. It does not imply that this is how the brain operates.
References:
Kant, I., Caygill, H., & Banham, G. (2007). Critique of pure reason (N. K. Smith, Trans.; Reissued edition). Palgrave Macmillan.
Clark, A. (2016). Surfing uncertainty: Prediction, action, and the embodied mind. Oxford University Press.
Hohwy, J. (2020). New directions in predictive processing. Mind & Language, 35. https://doi.org/10.1111/mila.12281
Morrot, G., Brochet, F., & Dubourdieu, D. (2001). The color of odors. Brain and Language, 79(2), 309–320. https://doi.org/10.1006/brln.2001.2493
Parr, T., Pezzulo, G., & Friston, K. J. (2022). Active inference: The free energy principle in mind, brain, and behavior. The MIT Press.
Rao, R. P., & Ballard, D. H. (1999). Predictive coding in the visual cortex: A functional interpretation of some extra-classical receptive-field effects. Nature Neuroscience, 2(1), 79–87. https://doi.org/10.1038/4580